Searching for "algorithms"
A Strategist’s Guide to Industry 4.0. Global businesses are about to integrate their operations into a seamless digital whole, and thereby change the world.
Industrial revolutions are momentous events. By most reckonings, there have been only three. The first was triggered in the 1700s by the commercial steam engine and the mechanical loom. The harnessing of electricity and mass production sparked the second, around the start of the 20th century. The computer set the third in motion after World War II.
Henning Kagermann, the head of the German National Academy of Science and Engineering (Acatech), did exactly that in 2011, when he used the term Industrie 4.0 to describe a proposed government-sponsored industrial initiative.
The term Industry 4.0 refers to the combination of several major innovations in digital technology
These technologies include advanced robotics and artificial intelligence; sophisticated sensors; cloud computing; the Internet of Things; data capture and analytics; digital fabrication (including 3D printing); software-as-a-service and other new marketing models; smartphones and other mobile devices; platforms that use algorithms to direct motor vehicles (including navigation tools, ride-sharing apps, delivery and ride services, and autonomous vehicles); and the embedding of all these elements in an interoperable global value chain, shared by many companies from many countries.
Companies that embrace Industry 4.0 are beginning to track everything they produce from cradle to grave, sending out upgrades for complex products after they are sold (in the same way that software has come to be updated). These companies are learning mass customization: the ability to make products in batches of one as inexpensively as they could make a mass-produced product in the 20th century, while fully tailoring the product to the specifications of the purchaser
.
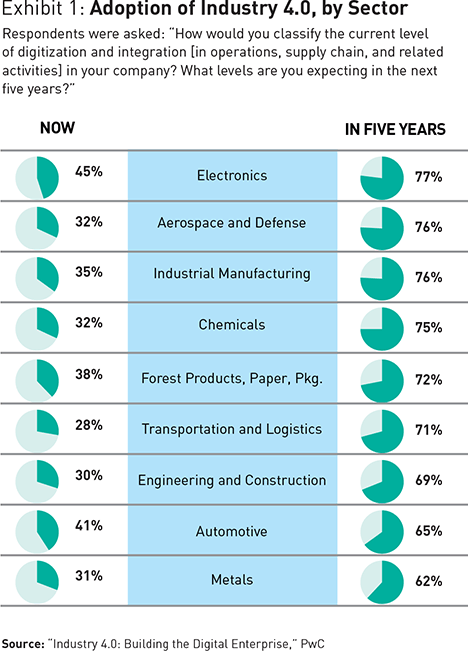
Three aspects of digitization form the heart of an Industry 4.0 approach.
• The full digitization of a company’s operations
• The redesign of products and services
• Closer interaction with customers
Making Industry 4.0 work requires major shifts in organizational practices and structures. These shifts include new forms of IT architecture and data management, new approaches to regulatory and tax compliance, new organizational structures, and — most importantly — a new digitally oriented culture, which must embrace data analytics as a core enterprise capability.
Klaus Schwab put it in his recent book The Fourth Industrial Revolution (World Economic Forum, 2016), “Contrary to the previous industrial revolutions, this one is evolving at an exponential rather than linear pace.… It is not only changing the ‘what’ and the ‘how’ of doing things, but also ‘who’ we are.”
This great integrating force is gaining strength at a time of political fragmentation — when many governments are considering making international trade more difficult. It may indeed become harder to move people and products across some national borders. But Industry 4.0 could overcome those barriers by enabling companies to transfer just their intellectual property, including their software, while letting each nation maintain its own manufacturing networks.
+++++++++++++++++++++++++++
more on the Internet of Things in this IMS blog
https://blog.stcloudstate.edu/ims?s=internet+of+things
also Digital Learning
https://blog.stcloudstate.edu/ims/2017/03/28/digital-learning/
Google Researchers Create AI That Builds Its Own Encryption
BY TOM BRANT OCTOBER 28, 2016 04:45PM EST
http://www.pcmag.com/news/349154/google-researchers-create-ai-that-builds-its-own-encryption
Alice and Bob have figured out a way to have a conversation without Eve being able to overhear, no matter how hard she tries.
They’re artificial intelligence algorithms created by Google engineers, and their ability to create an encryption protocol that Eve (also an AI algorithm) can’t hack is being hailed as an important advance in machine learning and cryptography.
Martin Abadi and David G. Andersen, explained in a paper published this week that their experiment is intended to find out if neural networks—the building blocks of AI—can learn to communicate secretly.
As the Abadi and Anderson wrote, “instead of training each of Alice and Bob separately to implement some known cryptosystem, we train Alice and Bob jointly to communicate successfully and to defeat Eve without a pre-specified notion of what cryptosystem they may discover for this purpose.”
same in German
Googles AI entwickelt eigenständig Verschlüsselung
Google-Forscher Martin Abadi und David G. Andersen des Deep-Learning-Projekts “Google Brain” eine neue Verschlüsselungsmethode entwickelt beziehungsweise entwickeln lassen. Die Forscher haben verschiedene neurale Netze damit beauftragt, eine abhörsichere Kommunikation aufzustellen.
++++++++++++++++
more on AI in this IMS blog:
https://blog.stcloudstate.edu/ims?s=artificial+intelligence
http://www.rubedo.com.br/2016/08/38-great-resources-for-learning-data.html
Learn data mining languages: R, Python and SQL
W3Schools – Fantastic set of interactive tutorials for learning different languages. Their SQL tutorial is second to none. You’ll learn how to manipulate data in MySQL, SQL Server, Access, Oracle, Sybase, DB2 and other database systems.
Treasure Data – The best way to learn is to work towards a goal. That’s what this helpful blog series is all about. You’ll learn SQL from scratch by following along with a simple, but common, data analysis scenario.
10 Queries – This course is recommended for the intermediate SQL-er who wants to brush up on his/her skills. It’s a series of 10 challenges coupled with forums and external videos to help you improve your SQL knowledge and understanding of the underlying principles.
TryR – Created by Code School, this interactive online tutorial system is designed to step you through R for statistics and data modeling. As you work through their seven modules, you’ll earn badges to track your progress helping you to stay on track.
Leada – If you’re a complete R novice, try Lead’s introduction to R. In their 1 hour 30 min course, they’ll cover installation, basic usage, common functions, data structures, and data types. They’ll even set you up with your own development environment in RStudio.
Advanced R – Once you’ve mastered the basics of R, bookmark this page. It’s a fantastically comprehensive style guide to using R. We should all strive to write beautiful code, and this resource (based on Google’s R style guide) is your key to that ideal.
Swirl – Learn R in R – a radical idea certainly. But that’s exactly what Swirl does. They’ll interactively teach you how to program in R and do some basic data science at your own pace. Right in the R console.
Python for beginners – The Python website actually has a pretty comprehensive and easy-to-follow set of tutorials. You can learn everything from installation to complex analyzes. It also gives you access to the Python community, who will be happy to answer your questions.
PythonSpot – A complete list of Python tutorials to take you from zero to Python hero. There are tutorials for beginners, intermediate and advanced learners.
Read all about it: data mining books
Data Jujitsu: The Art of Turning Data into Product – This free book by DJ Patil gives you a brief introduction to the complexity of data problems and how to approach them. He gives nice, understandable examples that cover the most important thought processes of data mining. It’s a great book for beginners but still interesting to the data mining expert. Plus, it’s free!
Data Mining: Concepts and Techniques – The third (and most recent) edition will give you an understanding of the theory and practice of discovering patterns in large data sets. Each chapter is a stand-alone guide to a particular topic, making it a good resource if you’re not into reading in sequence or you want to know about a particular topic.
Mining of Massive Datasets – Based on the Stanford Computer Science course, this book is often sighted by data scientists as one of the most helpful resources around. It’s designed at the undergraduate level with no formal prerequisites. It’s the next best thing to actually going to Stanford!
Hadoop: The Definitive Guide – As a data scientist, you will undoubtedly be asked about Hadoop. So you’d better know how it works. This comprehensive guide will teach you how to build and maintain reliable, scalable, distributed systems with Apache Hadoop. Make sure you get the most recent addition to keep up with this fast-changing service.
Online learning: data mining webinars and courses
DataCamp – Learn data mining from the comfort of your home with DataCamp’s online courses. They have free courses on R, Statistics, Data Manipulation, Dynamic Reporting, Large Data Sets and much more.
Coursera – Coursera brings you all the best University courses straight to your computer. Their online classes will teach you the fundamentals of interpreting data, performing analyzes and communicating insights. They have topics for beginners and advanced learners in Data Analysis, Machine Learning, Probability and Statistics and more.
Udemy – With a range of free and pay for data mining courses, you’re sure to find something you like on Udemy no matter your level. There are 395 in the area of data mining! All their courses are uploaded by other Udemy users meaning quality can fluctuate so make sure you read the reviews.
CodeSchool – These courses are handily organized into “Paths” based on the technology you want to learn. You can do everything from build a foundation in Git to take control of a data layer in SQL. Their engaging online videos will take you step-by-step through each lesson and their challenges will let you practice what you’ve learned in a controlled environment.
Udacity – Master a new skill or programming language with Udacity’s unique series of online courses and projects. Each class is developed by a Silicon Valley tech giant, so you know what your learning will be directly applicable to the real world.
Treehouse – Learn from experts in web design, coding, business and more. The video tutorials from Treehouse will teach you the basics and their quizzes and coding challenges will ensure the information sticks. And their UI is pretty easy on the eyes.
Learn from the best: top data miners to follow
John Foreman – Chief Data Scientist at MailChimp and author of Data Smart, John is worth a follow for his witty yet poignant tweets on data science.
DJ Patil – Author and Chief Data Scientist at The White House OSTP, DJ tweets everything you’ve ever wanted to know about data in politics.
Nate Silver – He’s Editor-in-Chief of FiveThirtyEight, a blog that uses data to analyze news stories in Politics, Sports, and Current Events.
Andrew Ng – As the Chief Data Scientist at Baidu, Andrew is responsible for some of the most groundbreaking developments in Machine Learning and Data Science.
Bernard Marr – He might know pretty much everything there is to know about Big Data.
Gregory Piatetsky – He’s the author of popular data science blog
KDNuggets, the leading newsletter on data mining and knowledge discovery.
Christian Rudder – As the Co-founder of OKCupid, Christian has access to one of the most unique datasets on the planet and he uses it to give fascinating insight into human nature, love, and relationships
Dean Abbott – He’s contributed to a number of data blogs and authored his own book on Applied Predictive Analytics. At the moment, Dean is Chief Data Scientist at
SmarterHQ.
Practice what you’ve learned: data mining competitions
Kaggle – This is the ultimate data mining competition. The world’s biggest corporations offer big prizes for solving their toughest data problems.
Stack Overflow – The best way to learn is to teach. Stackoverflow offers the perfect forum for you to prove your data mining know-how by answering fellow enthusiast’s questions.
TunedIT – With a live leaderboard and interactive participation, TunedIT offers a great platform to flex your data mining muscles.
DrivenData – You can find a number of nonprofit data mining challenges on DataDriven. All of your mining efforts will go towards a good cause.
Quora – Another great site to answer questions on just about everything. There are plenty of curious data lovers on there asking for help with data mining and data science.
Meet your fellow data miner: social networks, groups and meetups
Facebook – As with many social media platforms, Facebook is a great place to meet and interact with people who have similar interests. There are a number of very active data mining groups you can join.
LinkedIn – If you’re looking for data mining experts in a particular field, look no further than LinkedIn. There are hundreds of data mining groups ranging from the generic to the hyper-specific. In short, there’s sure to be something for everyone.
Meetup – Want to meet your fellow data miners in person? Attend a meetup! Just search for data mining in your city and you’re sure to find an awesome group near you.
——————————
8 fantastic examples of data storytelling
8 fantastic examples of data storytelling
Data storytelling is the realization of great data visualization. We’re seeing data that’s been analyzed well and presented in a way that someone who’s never even heard of data science can get it.
Google’s Cole Nussbaumer provides a friendly reminder of what data storytelling actually is, it’s straightforward, strategic, elegant, and simple.
++++++++++++++++++++++
more on text and data mining in this IMS blog
hthttps://blog.stcloudstate.edu/ims?s=data+mining
The biggest threat to democracy? Your social media feed
Yochai Benkler explains: “The various formats of the networked public sphere provide anyone with an outlet to speak, to inquire, to investigate, without need to access the resources of a major media organization.”
Democratic bodies are typically elected in periods of three to five years, yet citizen opinions seem to fluctuate daily and sometimes these mood swings grow to enormous proportions. When thousands of people all start tweeting about the same subject on the same day, you know that something is up. With so much dynamic and salient political diversity in the electorate, how can policy-makers ever reach a consensus that could satisfy everyone?
At the same time, it would be a grave mistake to discount the
voices of the internet as something that has no connection to real political situations.
What happened in the UK was not only a political disaster, but also a vivid example of what happens when you combine the uncontrollable power of the internet with a
lingering visceral feeling that ordinary people have lost control of the politics that shape their lives.
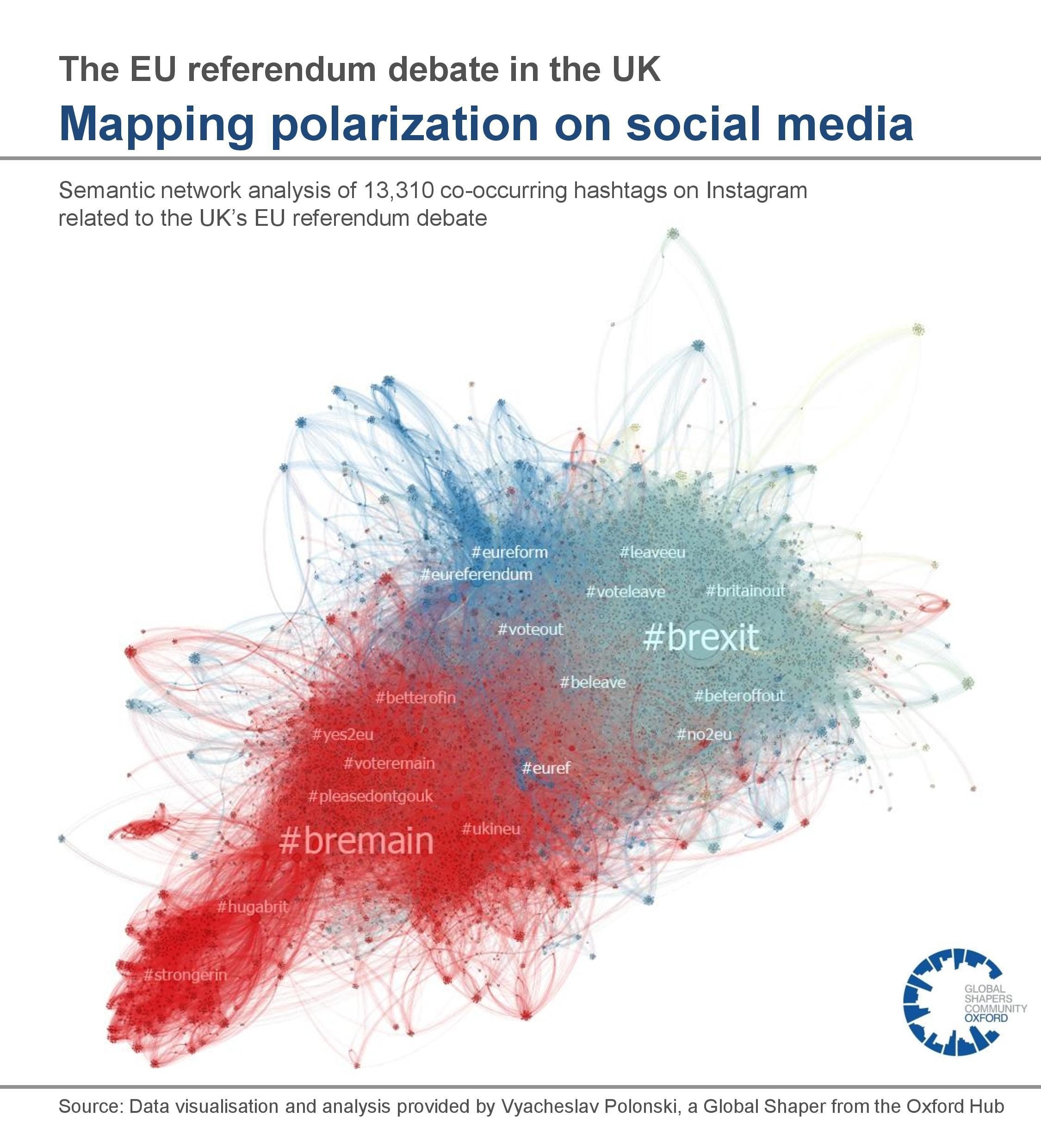
Polarization as a driver of populism
People who have long entertained right-wing populist ideas, but were never confident enough to voice them openly, are now in a position to connect to like-minded others online and use the internet as a megaphone for their opinions.
The resulting echo chambers tend to amplify and reinforce our existing opinions, which is dysfunctional for a healthy democratic discourse. And while social media platforms like Facebook and Twitter generally have the power to expose us to politically diverse opinions, research suggests that the filter bubbles they sometimes create are, in fact, exacerbated by the platforms’ personalization algorithms, which are based on our social networks and our previously expressed ideas. This means that instead of creating an ideal type of a digitally mediated “public agora”, which would allow citizens to voice their concerns and share their hopes, the internet has actually increased conflict and ideological segregation between opposing views, granting a disproportionate amount of clout to the most extreme opinions.
The disintegration of the general will
In political philosophy, the very idea of democracy is based on the principal of the general will, which was proposed by Jean-Jacques Rousseau in the 18th century. Rousseau envisioned that a society needs to be governed by a democratic body that acts according to the imperative will of the people as a whole.
There can be no doubt that a new form of digitally mediated politics is a crucial component of the Fourth Industrial Revolution: the internet is already used for bottom-up agenda-setting, empowering citizens to speak up in a networked public sphere, and pushing the boundaries of the size, sophistication and scope of collective action. In particular, social media has changed the nature of political campaigning and will continue to play an important role in future elections and political campaigns around the world.
++++++++++++++
more on the impact of technology on democracy in this IMS blog:
https://blog.stcloudstate.edu/ims?s=democracy
Security Tops List of Trends That Will Impact the Internet of Things
By David Nage 02/25/16
https://campustechnology.com/articles/2016/02/25/security-tops-list-of-trends-that-will-impact-the-internet-of-things.aspx
Are you ready to deal with “denial of sleep” attacks? Those are attacks using malicious code, propagated through the Internet of Things, aimed at draining the batteries of your devices by keeping them awake.
- Security. threats extend well beyond denial of sleep: “The IoT introduces a wide range of new security risks and challenges to the IoT devices themselves, their platforms and operating systems, their communications, and even the systems to which they’re connected.
- Analytics. IoT will require a new approach to analytics. “New analytic tools and algorithms are needed now, but as data volumes increase through 2021, the needs of the IoT may diverge further from traditional analytics,” according to Gartner.
- Device (Thing) Management. IoT things that are not ephemeral — that will be around for a while — will require management like every other device (firmware updates, software updates, etc.), and that introduces problems of scale.
- Low-Power, Short-Range IoT Networks. Short-range networks connecting IT devices will be convoluted. There will not be a single common infrastructure connecting devices.
- Low-Power, Wide-Area Networks. Current solutions are proprietary, but standards will come to dominate.
- Processors and Architecture. Designing devices with an understanding of those devices’ needs will require “deep technical skills.”
- Operating Systems. There’s a wide range of systems out there that have been designed for specific purposes.
- Event Stream Processing. “Some IoT applications will generate extremely high data rates that must be analyzed in real time.
- Platforms. “IoT platforms bundle many of the infrastructure components of an IoT system into a single product.
- Standards and Ecosystems. as IoT devices proliferate, new ecosystems will emerge, and there will be “commercial and technical battles between these ecosystems” that “will dominate areas such as the smart home, the smart city and healthcare.
Iran’s blogfather: Facebook, Instagram and Twitter are killing the web
http://www.theguardian.com/technology/2015/dec/29/irans-blogfather-facebook-instagram-and-twitter-are-killing-the-web
is it possible that the Iranian government realized the evolution of social media and his respective obsolescence and this is why they freed him prematurely?
Blogs were gold and bloggers were rock stars back in 2008 when I was arrested.
The hyperlink was a way to abandon centralisation – all the links, lines and hierarchies – and replace them with something more distributed, a system of nodes and networks. Since I got out of jail, though, I’ve realised how much the hyperlink has been devalued, almost made obsolete.
Nearly every social network now treats a link as just the same as it treats any other object – the same as a photo, or a piece of text. You’re encouraged to post one single hyperlink and expose it to a quasi-democratic process of liking and plussing and hearting. But links are not objects, they are relations between objects. This objectivisation has stripped hyperlinks of their immense powers.
At the same time, these social networks tend to treat native text and pictures – things that are directly posted to them – with a lot more respect. One photographer friend explained to me how the images he uploads directly to Facebook receive many more likes than when he uploads them elsewhere and shares the link on Facebook.
Some networks, like Twitter, treat hyperlinks a little better. Others are far more paranoid. Instagram – owned by Facebook – doesn’t allow its audiences to leave whatsoever. You can put up a web address alongside your photos, but it won’t go anywhere. Lots of people start their daily online routine in these cul-de-sacs of social media, and their journeys end there. Many don’t even realise they are using the internet’s infrastructure when they like an Instagram photograph or leave a comment on a friend’s Facebook video. It’s just an app.
A most brilliant paragraph by some ordinary-looking person can be left outside the stream, while the silly ramblings of a celebrity gain instant internet presence. And not only do the algorithms behind the stream equate newness and popularity with importance, they also tend to show us more of what we have already liked. These services carefully scan our behaviour and delicately tailor our news feeds with posts, pictures and videos that they think we would most likely want to see.
Today the stream is digital media’s dominant form of organising information. It’s in every social network and mobile application.
The centralisation of information also worries me because it makes it easier for things to disappear.
But the scariest outcome of the centralisation of information in the age of social networks is something else: it is making us all much less powerful in relation to governments and corporations. Surveillance is increasingly imposed on civilised lives, and it gets worse as time goes by. The only way to stay outside of this vast apparatus of surveillance might be to go into a cave and sleep, even if you can’t make it 300 years.
Center for Digital Education (CDE)
real-time impact on curriculum structure, instruction delivery and student learning, permitting change and improvement. It can also provide insight into important trends that affect present and future resource needs.
Big Data: Traditionally described as high-volume, high-velocity and high-variety information.
Learning or Data Analytics: The measurement, collection, analysis and reporting of data about learners and their contexts, for purposes of understanding and optimizing learning and the environments in which it occurs.
Educational Data Mining: The techniques, tools and research designed for automatically extracting meaning from large repositories of data generated by or related to people’s learning activities in educational settings.
Predictive Analytics: Algorithms that help analysts predict behavior or events based on data.
Predictive Modeling: The process of creating, testing and validating a model to best predict the probability of an outcome.
Data analytics, or the measurement, collection, analysis and reporting of data, is driving decisionmaking in many institutions. However, because of the unique nature of each district’s or college’s data needs, many are building their own solutions.
For example, in 2014 the nonprofit company inBloom, Inc., backed by $100 million from the Gates Foundation and the Carnegie Foundation for the Advancement of Teaching, closed its doors amid controversy regarding its plan to store, clean and aggregate a range of student information for states and districts and then make the data available to district-approved third parties to develop tools and dashboards so the data could be used by classroom educators.22
Tips for Student Data Privacy
Know the Laws and Regulations
There are many regulations on the books intended to protect student privacy and safety: the Family Educational Rights and Privacy Act (FERPA), the Protection of Pupil Rights Amendment (PPRA), the Children’s Internet Protection Act (CIPA), the Children’s Online Privacy Protection Act (COPPA) and the Health Insurance Portability and Accountability Act (HIPAA)
— as well as state, district and community laws. Because technology changes so rapidly, it is unlikely laws and regulations will keep pace with new data protection needs. Establish a committee to ascertain your institution’s level of understanding of and compliance with these laws, along with additional safeguard measures.
Make a Checklist Your institution’s privacy policies should cover security, user safety, communications, social media, access, identification rules, and intrusion detection and prevention.
Include Experts
To nail down compliance and stave off liability issues, consider tapping those who protect privacy for a living, such as your school attorney, IT professionals and security assessment vendors. Let them review your campus or district technologies as well as devices brought to campus by students, staff and instructors. Finally, a review of your privacy and security policies, terms of use and contract language is a good idea.
Communicate, Communicate, Communicate
Students, staff, faculty and parents all need to know their rights and responsibilities regarding data privacy. Convey your technology plans, policies and requirements and then assess and re-communicate those throughout each year.
“Anything-as-a-Service” or “X-as-a-Service” solutions can help K-12 and higher education institutions cope with big data by offering storage, analytics capabilities and more. These include:
• Infrastructure-as-a-Service (IaaS): Providers offer cloud-based storage, similar to a campus storage area network (SAN)
• Platform-as-a-Service (PaaS): Opens up application platforms — as opposed to the applications themselves — so others can build their own applications
using underlying operating systems, data models and databases; pre-built application components and interfaces
• Software-as-a-Service (SaaS): The hosting of applications in the cloud
• Big-Data-as-a-Service (BDaaS): Mix all the above together, upscale the amount of data involved by an enormous amount and you’ve got BDaaS
Suggestions:
Use accurate data correctly
Define goals and develop metrics
Eliminate silos, integrate data
Remember, intelligence is the goal
Maintain a robust, supportive enterprise infrastructure.
Prioritize student privacy
Develop bullet-proof data governance guidelines
Create a culture of collaboration and sharing, not compliance.
more on big data in this IMS blog:
https://blog.stcloudstate.edu/ims/?s=big+data&submit=Search
Response: Personalized Learning Is ‘a Partnership With Students’
http://blogs.edweek.org/teachers/classroom_qa_with_larry_ferlazzo/2015/09/response_personalized_learning_is_a_learning_partnership_with_students.html
building relationships with students so I can better connect lessons to their interests, hopes and dreams; providing them with many opportunities for organizational and cognitive choice; and creating situations where they can get positive, as well as critical, feedback in a supportive way from me, their classmates and themselves.
Response: Personalized Learning Is ‘Based On Relationships, Not Algorithms’
http://blogs.edweek.org/teachers/classroom_qa_with_larry_ferlazzo/2015/09/response_personalized_learning_is_based_on_relationships_not_algorithms.html
Too often, the notion of “personalized learning” means choice-based programmed rather than truly personalized. This comes from the tech world, where “personalization” is synonymous with user choice. It’s the idea of giving a thumbs up or a thumbs down on Pandora. It’s the idea of having adaptive programs that change based upon one’s personal preferences. It’s the Facebook algorithm that tells you what information is the most relevant to you. It’s about content delivery rather than user creation.
While tech companies promise personalization, they often promote independent, isolated learning. True personalization is interdependent rather than isolated. True personalization is based upon a horizontal relationship rather than a top-down customization. True personalization is based upon a deeply human relationship rather than a program or an algorithm or a set of scripts. True personalization is a mix between personal autonomy and group belonging. It’s a mix between what someone wants and what someone needs. It’s a chance to make, rather than simply a chance to consume.